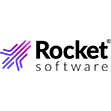
Blowing Up Silos In a Big Data World
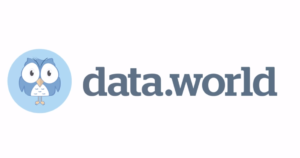
A startup named data.world is embarking upon a grand experiment to build a collaborative data platform that links together data, people, and their analytic tools. By eradicating data silos and building a social community around data, the firm is betting that it can grease the wheels on insight discovery and unleash a network effect on data.
“The world is one giant map of data, things happening all the time, people, places, things, and if you shrink that world and make it much easier to understand, you’re going to find correlations between things you never knew were correlated,” says Brett Hurt, data.world‘s co-founder and CEO. “We know that that’s going to be the way we solve things like cancer and climate change and other big problems in the world. But the longer that we stay siloed and in in our own little private data world, the less effective we’re all going to be.”
You certainly can’t accuse Hurt, a serial entrepreneur now on his sixth startup, of thinking small. Having previously founded companies like Web analytics firm Coremetrics, which was acquired by IBM, and bazaarvoice, which had a $1 billion IPO, Hurt was looking for a bigger problem to tackle. The disconnectedness of data seemed like a good one, so he joined forces with three other technologists from vacation rental firm HomeAway — Matt Laessig, Jon Loyens, and Bryon Jacob – and founded data.world in 2015.
Three years and $32.7 million in funding later, the Austin, Texas firm has enjoyed a fair bit of success, with users all over the world. With today’s launch of an enterprise version of the product, data.world is on the cusp of spreading its mantra of mutual data connectedness deep behind the corporate firewall.
Bridging Worlds
From a practical point of view, the data.world platform resembles other products that you can find practicing data scientists and data analysts using every day.
The manner in which users can import all types of data into the platform, and how the platform then catalogs and tracks the data, puts it in a category alongside data catalog and data discovery tools. Once the data has been imported into the AWS-based service, it can do some quick analysis of the data, such as showing the users minimums, maximums, means, and standard deviations, not unlike a data quality tool.
The manner in which the data.world platform tracks all actions made against the data and keeps a detailed lineage of transformations to raw data and the derived data that results resembles some metadata management tools. And the way that the platform lets users collaborate and share data and ideas isn’t unlike how some data science platforms operate.
The data.world folks don’t disagree that the platform does things that other tools can do. But they argue that the whole is more than the sum of its parts, and one of the key ways that is true is from its use of semantic Web technology.
“When you load a piece of data in, whether it’s a piece of open data or private data set, we actually convert it to semantically linkable graph data,” Loyens tells Datanami. “Essentially we are a giant graph database as a service. What that really means is we maintain a social graph of you, your colleagues, and who you’re working with and what you’re working on.”
That graph aspect of data.world is the key to tracking the capture of data and what people do with it as they create knowledge. And once that knowledge is created, it’s logged back into the platform to help the next person who comes along, who now has a recipe for how that derived data was concocted.
The problem is that “knowledge management” is a dirty word to many in the big data space. “At the end of the day, a lot of data scientists and data analysts and researches dislike doing knowledge management,” Loyens says. “It’s an extra chore. Why MDM processes end up being big enterprise-y things is because all of a sudden we have to do all this extra documentation, extra work, to make your stuff usable.
“I like to joke,” he continues, “that we’re a knowledge management platform disguised as a social network, because a lot of people like participating in social things. We’re trying to replicate that water cooler conversation with that spreadsheet or temporal data set that goes around, but we try to do it in a way that we can reuse that data later on.”
From the Web browser interface, data.world lets users bookmark they’re favorite data sets. And because the data is all stored in a semantic graph database, users can search for it with SPARQL queries, or even run standard SQL queries against it.
Grow from the Middle
For all that it has on its plate, the folks at data.world seem to be conscious about the importance of not trying to do too much. Instead of developing BI and SQL reporting tools to make the data analysis of data in the platform really pop, it partnered with Tableau and Microsoft for PowerBI. Microstrategy used the open data.world API to build its own integration without any help from data.world.
The company is also working with IBM to hook SPSS into the platform, and has a partnership with machine learning marketplace provider Algorithmia for running machine learning workloads on the cloud (data.world doesn’t supply data processing capabilities at this time). Users can also bring numerous Python and R-based data science tools and data science notebooks to bear on the data they have stored in data.world.
“We view ourselves very much like a neutral party that sits in the middle and this is where you do collaboration, this is where you find and discover data inside your organization, this is where you can clean that up, make it well documented, make everybody able to work with it much more efficiently, leading to that increase in productivity,” Hurt says. “But I don’t want to be disconnected from that world of analytic tools and machine learning tools and everything else that you use. That’s why those integrations are so important. That makes us unique. We really sit in the middle of the toolchains.”
Being in the middle allows data scientists to quickly ping subject matter experts to ask what the column for “revenue” means, gross or net? “Those actually have big implications to business decisions,” Loyens says, but the process that companies often have for answering those questions “often resembles a schoolyard game of telephone, where really important knowledge will get dropped off along the way. We think we’ve layered in a layer of data centricity to all this that hasn’t existed before.”
The company is consciously trying to replicate the success of open source software development on the world of data. While data and software are admittedly two different things, the core similarity that matters here is the push to be open. When more data is shared in an open manner, it can lead to new correlations, new insights, new business models, and value that didn’t exist before.
“Our overarching mission statement is to build the world’s most collaborative, abundant, and meaningful data resource,” Hurt says. “The reality is we live in this networked age and for whatever reasons, data hasn’t been easy to work with and it’s been largely disconnected outside of, say, online advertising data. There’s so much data inside corporations, so much open data in the world to bring to bear to solve all types of problems. We’re very mission driven in wiring all that up in this space.”
Related Items:
Why 2018 Will Be All About the Data
Five Tips for Winning at Data Governance