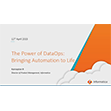
ChatGPT Gives Kinetica a Natural Language Interface for Speedy Analytics Database
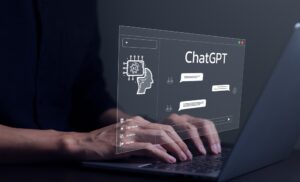
(SuPatMaN/Shutterstock)
It would normally take quite a bit of complex SQL to tease a multi-pronged answer out of Kinetica’s high-speed analytics database, which is powered by GPUs but wire-compataible with Postgres. But with the new natural language interface to ChatGPT unveiled today, non-technical users can get answers to complex questions written in plain English.
Kinetica was incubated by the U.S. Army over a decade ago to pour through huge mounds of fast-moving geospatial and temporal data in search of terrorist activity. By leveraging the processing capability of GPUs, the vector database could run full table scans on the data, whereas other databases were forced to winnow down the data with indexes and other techniques (it has since embraced CPUs with Intel’s AVX-512).
With today’s launch of its new Conversational Query feature, Kinetica’s massive processing capability is now within the reach of workers who lack the ability to write complex SQL queries. That democratization of access means executives and others with ad-hoc data questions are now able to leverage the power of Kinetica’s database to get answers.
The vast majority of database queries are planned, which enables organizations to write indexes, de-normalize the data, or pre-compute aggregates to get those queries to run in a performant way, says Kinetica co-founder and CEO Nima Negahban.
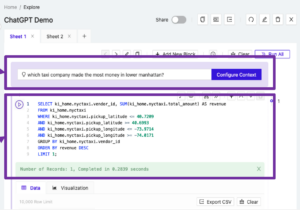
A user can submit a natural langauge query directly on the Kinetica dashboard, which ChatGPT converts to SQL for execution
“With the advent of generative large language models, we think that that mix is going to change to where a lot bigger portion of it’s going be ad hoc queries,” Negahban tells Datanami. “That’s really what we do best, is do that ad hoc, complex query against large datasets, because we have that ability to do large scans and leverage many-core compute devices better than other databases.”
Conversational Query works by converting a user’s natural language query into SQL. That SQL conversion is handled by OpenAI’s ChatGPT large language model (LLM), which proven itself to be a quick learner of language–spoken, computer, and otherwise. OpenAI API then returns the finalized SQL, and users can then choose to execute it against the database directly from the Kinetica dashboard.
Kinetica is leaning on the ChatGPT model to understand the intent of language, which is something that it’s very good at. For example, to answer the question “Where do people hang out the most?” from a massive database of geospatial data of human movement, ChatGPT is smart enough to know that “hang out” is a synonym for “dwell time,” which is how the data is officially identified in the database. (The answer, by the way, is 7-Eleven.)
Kinetica is also doing some work ahead of time to prepare ChatGPT to generate good SQL through its “hydration” process, says Chad Meley, Kinetica’s chief marketing officer.
“We have native analytic functions that are callable through SQL and ChatGPT, through part of the hydration process, becomes aware of that,” Meley says. “So it can use a specific time-series join or spatial join that we make ChatGPT aware of. In that way, we go beyond your typical ANSI SQL functions.”
The SQL generated by ChatGPT isn’t perfect. As many are aware, the LLM is prone to seeing things in the data, the so-called “hallucination” problem. But even though it’s SQL isn’t completely free of defect, ChatGPT is still quite useful at this state, says Negahban, who was a 2018 Datanami Person to Watch.
“I’ve seen that it’s kind of good enough,” he says. “It hasn’t been [wildly] wrong in any queries it generates…I think it will be better with GPT-4.”
In the end analysis, by the time it takes a SQL pro to write the perfect seven-way join and get it over to the database, the opportunity to act on the data may be gone. That’s why the pairing of a “good enough” query generator with a database as powerful as Kinetica can make a different for decision-makers, Negahban says.
“Having an engine like Kinetica that can actually do something with that query without having to do planning beforehand” is the big get, he says. “If you try to do some of these queries with the Snowflake, or insert your database du jour, they really struggle because that’s just not what they’re built for. They’re good at other things. What we’re really good at, as an engine, is to do ad hoc queries no matter the complexity, no matter how many tables are involved. So that really pairs well with this ability for anyone to generate SQL across all their data asking questions about all the data in their enterprise.”
Conversational Query is available now in the cloud and on-prem versions of Kinetica.
Related Items:
ChatGPT Dominates as Top In-Demand Workplace Skill: Udemy Report
Bank Replaces Hundreds of Spark Streaming Nodes with Kinetica
Preventing the Next 9/11 Goal of NORAD’s New Streaming Data Warehouse
April 24, 2025
- Dataiku Expands Platform with Tools to Build, Govern, and Monitor AI Agents at Scale
- Indicium Launches IndiMesh to Streamline Enterprise AI and Data Systems
- StorONE and Phison Unveil Storage Platform Designed for LLM Training and AI Workflows
- Dataminr Raises $100M to Accelerate Global Push for Real-Time AI Intelligence
- Elastic Announces General Availability of Elastic Cloud Serverless on Google Cloud Marketplace
- CNCF Announces Schedule for OpenTelemetry Community Day
- Thoughtworks Signs Global Strategic Collaboration Agreement with AWS
April 23, 2025
- Metomic Introduces AI Data Protection Solution Amid Rising Concerns Over Sensitive Data Exposure in AI Tools
- Astronomer Unveils Apache Airflow 3 to Power AI and Real-Time Data Workflows
- CNCF Announces OpenObservabilityCon North America
- Domino Wins $16.5M DOD Award to Power Navy AI Infrastructure for Mine Detection
- Endor Labs Raises $93M to Expand AI-Powered AppSec Platform
- Ocient Announces Close of Series B Extension Financing to Accelerate Solutions for Complex Data and AI Workloads
April 22, 2025
- O’Reilly Launches AI Codecon, New Virtual Conference Series on the Future of AI-Enabled Development
- Qlik Powers Alpha Auto Group’s Global Growth with Automotive-Focused Analytics
- Docker Extends AI Momentum with MCP Tools Built for Developers
- John Snow Labs Unveils End-to-End HCC Coding Solution at Healthcare NLP Summit
- PingCAP Expands TiDB with Vector Search, Multi-Cloud Support for AI Workloads
- Qumulo Launches New Pricing in AWS Marketplace
April 21, 2025
- PayPal Feeds the DL Beast with Huge Vault of Fraud Data
- OpenTelemetry Is Too Complicated, VictoriaMetrics Says
- Will Model Context Protocol (MCP) Become the Standard for Agentic AI?
- Thriving in the Second Wave of Big Data Modernization
- What Benchmarks Say About Agentic AI’s Coding Potential
- Google Cloud Preps for Agentic AI Era with ‘Ironwood’ TPU, New Models and Software
- Google Cloud Fleshes Out its Databases at Next 2025, with an Eye to AI
- Can We Learn to Live with AI Hallucinations?
- Monte Carlo Brings AI Agents Into the Data Observability Fold
- AI Today and Tomorrow Series #3: HPC and AI—When Worlds Converge/Collide
- More Features…
- Grafana’s Annual Report Uncovers Key Insights into the Future of Observability
- Google Cloud Cranks Up the Analytics at Next 2025
- New Intel CEO Lip-Bu Tan Promises Return to Engineering Innovation in Major Address
- AI One Emerges from Stealth to “End the Data Lake Era”
- SnapLogic Connects the Dots Between Agents, APIs, and Work AI
- Snowflake Bolsters Support for Apache Iceberg Tables
- GigaOM Report Highlights Top Performers in Unstructured Data Management for 2025
- Supabase’s $200M Raise Signals Big Ambitions
- New Benchmark for Real-Time Analytics Released by Timescale
- Big Data Career Notes for March 2025
- More News In Brief…
- Gartner Predicts 40% of Generative AI Solutions Will Be Multimodal By 2027
- MinIO: Introducing Model Context Protocol Server for MinIO AIStor
- Dataiku Achieves AWS Generative AI Competency
- AMD Powers New Google Cloud C4D and H4D VMs with 5th Gen EPYC CPUs
- Prophecy Introduces Fully Governed Self-Service Data Preparation for Databricks SQL
- Seagate Unveils IronWolf Pro 24TB Hard Drive for SMBs and Enterprises
- CData Launches Microsoft Fabric Integration Accelerator
- MLCommons Releases New MLPerf Inference v5.0 Benchmark Results
- Opsera Raises $20M to Expand AI-Driven DevOps Platform
- GitLab Announces the General Availability of GitLab Duo with Amazon Q
- More This Just In…